Overview of Enterprise Data Governance
Data governance consists of policies, processes and an organizational structure to support enterprise data management.
With the exponential growth of new data, data governance is no longer an essential component from compliance, but rather improves efficiency of data asset value and data driven processes.
In Todays world, self service capabilities are provided to business users for Data analytics and Data Science, Machine Learning platforms . Its is critical to have effective governance process for meeting Data quality standards, corporate security standards, regulatory controls, ease of data use.
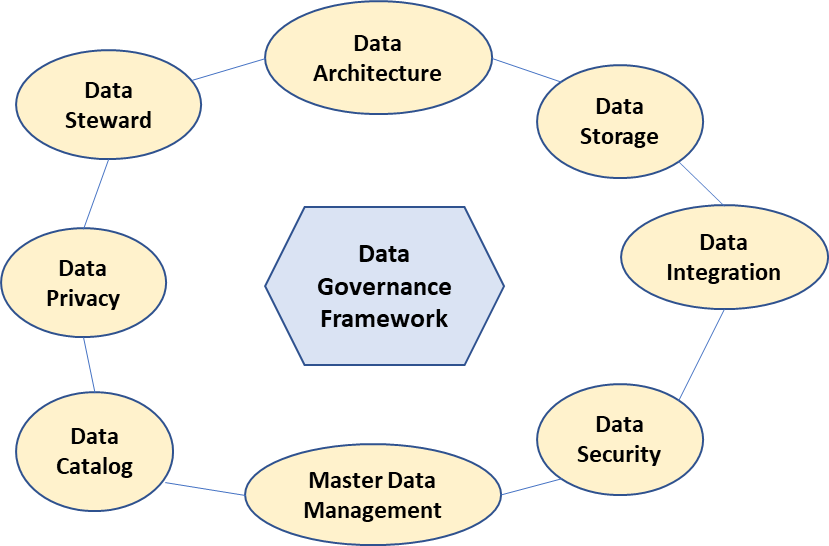
Data Governance Framework
Data Governance Framework comprises of Data Discovery, Transformation, Data quality, data catalog, data security and privacy, data lineage tracking, and data life cycle management components.
Data Discovery and Access
This step involves
- Identify the Data sources
- Data ingestion from from reliable sources
- Data Profiling
- Data Policy association
- Data Ingestion and Policy enforcement
Some of the categories where policies are required – Data quality, Data Compliance, Data Classification, Data Retention
Data Transformation
Data is available in many ways i.e. structured, unstructured and in multiple formats i.e. csv, parquet. Also, apart from conventional transformational tools, business users are rapidly performing self service transformation utilizing auto transformation, embedded ML capabilities of new ETL Platforms.
From Governance, all the Data transformation must be integrated with Data lineage tools. In case of Data lakes, define multiple Zones (Raw/Curated/Consumption) to assist in the data flow governance.
Data Catalog
Data catalog tools should be able to extract entities from Semi-structured and unstructured data to build semantic metadata and to be able to identify relationships between entities.
Data catalog is the key component for Self service Analytics . Implementing a highly governed Data catalog will help in discovering the data assets, understand data assets, Centralized access layer. Data catalog helps in providing comprehensive view of data, ease of use in data discovery, guardrails on data governance.
Implement auto crawlers & ML for data discovery and auto catalog tools. Tools like AWS Glue, Azure Purview will perform some of the auto catalog functions.
Ensure Metadata persisted , indexed and made available for end users.
Data Security
Data Security can be broadly split in to following three areas
- Authentication: Determining the legitimacy of the identity of a user
- Authorization: The privileges that a user holds to perform specific actions
- Access: The security mechanisms used to protect data, both in transit and at rest
Data Governance/Life Cycle Management
This step involves Operationalizing the analytical reports and ML Models through automation. Ensure end to end lineage is implemented for auditing and impact analysis
Due to constant changes in data, check for Data Drift, Schema Drift & Compliance Drift.
Define strategy and policies for classifying which data is valuable and how long you should store a particular dataset. The life span of data can be partitioned into multiple phases.
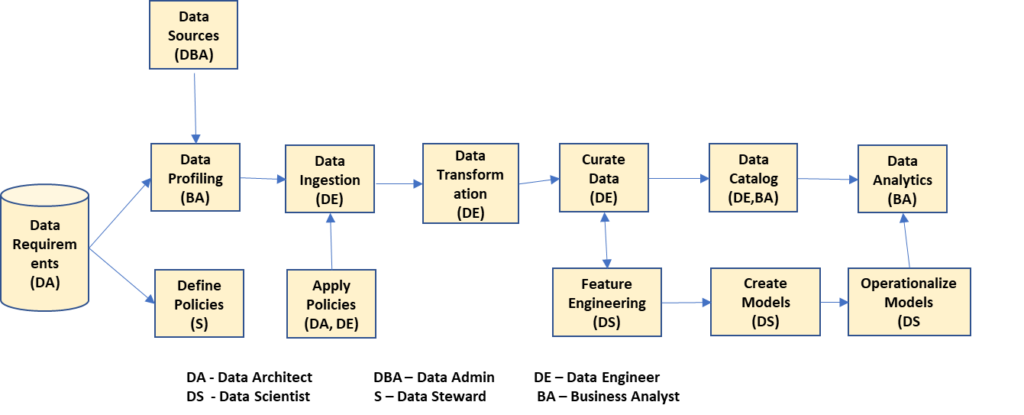
Data Quality Governance
Some of the Data quality Characteristics are
- Correctness/accuracy
- Completeness/coverage
- Consistency
- Timeliness
- Data lineage
Data quality standards involves
- Data Quality detection and remediation
- Check for null/missing values, duplicate values, extra characters
- Data Profiling and Classification
- Profile the data. Classify the data (PII/PHI/Confidential)
- Automate Data quality checks and remediation during Pipe line orchestration
- Monitor the Data quality by building and implementing the rules
- Notify the anomalies
- Remediate the issues in Source system
Data Governance Solutions Scoreboard
Forrester Data Governance Solutions Scoreboard as of Q3 2021
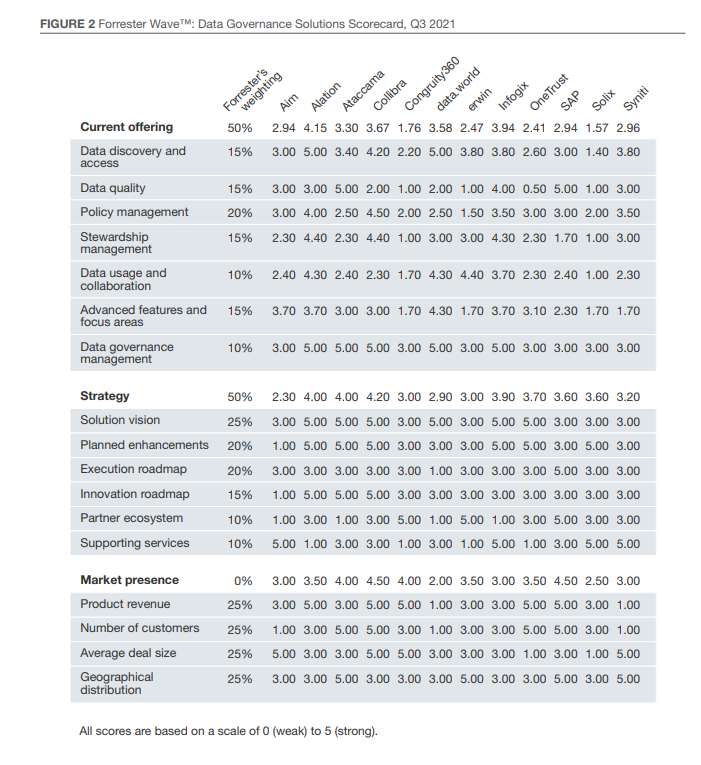